RAG for Creating Enterprise Website Content: Revolutionizing Content Creation with AI/ML
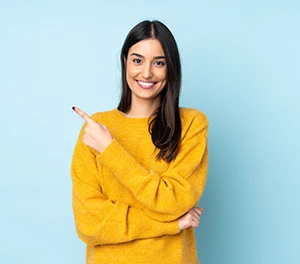
Amanda Jones
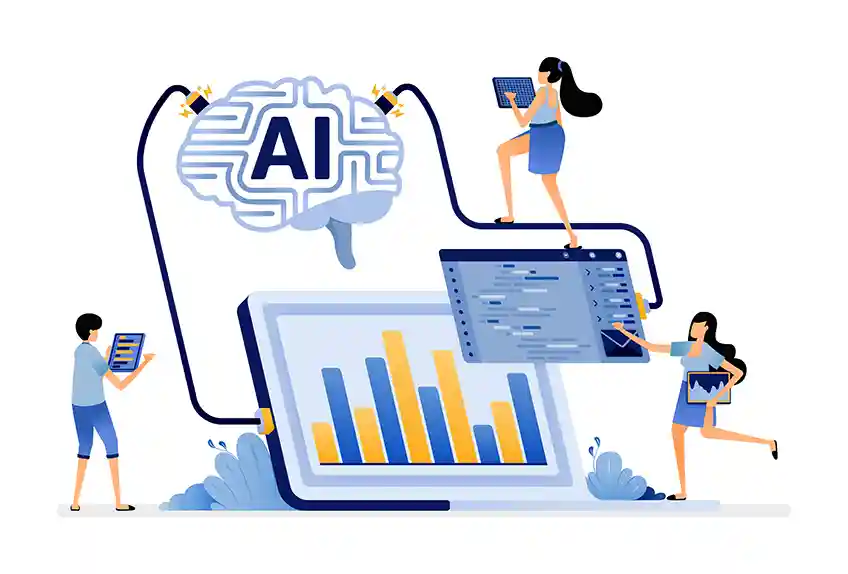
In the digital age, a compelling and dynamic online presence is crucial for enterprises. Creating high-quality, engaging content that resonates with the audience is a significant challenge. Retrieval-Augmented Generation (RAG) offers a groundbreaking solution to this challenge by combining the strengths of retrieval-based and generative models to enhance the content creation process. This blog post explores how RAG can be used to create enterprise website content, its benefits, and practical applications.
Understanding RAG: The Basics
What is RAG?
Retrieval-Augmented Generation (RAG) is a machine learning framework that integrates two key components of natural language processing (NLP): retrieval-based models and generative models. The retrieval-based component searches a large database of documents to find relevant information, while the generative component uses this information to produce coherent and contextually appropriate responses or content. This combination leverages the precision of retrieval systems with the creativity and fluidity of generative models, and significantly improves the accuracy of generative AI content creation.
Why RAG Matters for Enterprises
Traditional content creation methods can be time-consuming and often lack the ability to quickly adapt to changing market demands. RAG addresses these issues by ensuring that the generated content is both accurate and contextually relevant. This approach is particularly beneficial for enterprises that need to maintain a consistent and engaging online presence across various platforms and audience segments.
How RAG Works for Content Creation
The Architecture of RAG
A RAG system for content creation consists of two main components:
-
Retriever: This component searches a pre-existing database or corpus of documents to find the most relevant pieces of information related to the input query. The retriever can be based on various models, such as BM25, Dense Passage Retrieval (DPR), or other advanced information retrieval techniques.
-
Generator: Once the retriever identifies relevant documents, the generator uses this information to create a response. The generator is typically a neural network model, such as a transformer, that can synthesize the retrieved information into coherent and contextually appropriate text.
The Process
The process of using RAG for content creation involves several steps:
- Query Input: The system receives a query or prompt from the user, which could be a topic or a specific content requirement.
- Document Retrieval: The retriever searches the database for documents that are relevant to the query.
- Contextualization: The retrieved documents provide context for the generative model.
- Content Generation: The generative model produces content based on the contextual information.
- Output: The final output is content that is both accurate and contextually rich, combining the best elements of retrieval and generation.
Applications of RAG in Enterprise Web Content Creation
Enhanced Website Content
One of the primary applications of RAG in enterprises is enhancing website content. Whether it's blog posts, product descriptions, or landing pages, RAG can help generate content that is informative, engaging, and tailored to the target audience. For example, a company can input a query related to a new product launch, and the RAG system can retrieve relevant information from internal databases and generate a detailed product description.
Personalized User Experiences
RAG can be used to create personalized user experiences by generating content tailored to individual user preferences. For instance, an e-commerce website can use RAG to generate personalized product recommendations and descriptions based on a user's browsing history and preferences. This level of personalization can significantly enhance user engagement and drive conversions.
Dynamic FAQ Sections
Maintaining an up-to-date FAQ section on a website can be challenging, especially for large enterprises with a wide range of products and services. RAG can automate this process by retrieving relevant information from a database of past customer queries and generating accurate and contextually relevant responses. This not only saves time but also ensures that the information provided is always current.
Content Localization
Enterprises operating in multiple regions often need to create localized content to cater to different cultural and linguistic contexts. RAG can assist in this process by retrieving relevant information about local markets and generating content that is appropriately localized. This can include translating content into different languages and adapting it to local cultural norms and preferences.
Benefits of RAG for Enterprise Web Content Creation
Improved Efficiency
RAG significantly improves the efficiency of content creation by automating the retrieval and generation processes. This allows content creators to focus on higher-level tasks such as strategy and creativity, while the RAG system handles the more routine aspects of content production.
Consistency and Accuracy
By leveraging a vast database of information, RAG ensures that the generated content is both accurate and consistent with the enterprise's brand voice and messaging. This is particularly important for large organizations that need to maintain a consistent online presence across multiple platforms and regions.
Scalability
RAG systems can scale to handle large volumes of content creation tasks, making them suitable for enterprises with extensive content requirements. Whether it's generating thousands of product descriptions or creating personalized content for millions of users, RAG can efficiently manage the workload.
Flexibility
RAG systems are highly flexible and can be adapted to a wide range of content creation tasks. Whether it's generating technical documentation, marketing materials, or customer support content, RAG can be tailored to meet the specific needs of different departments and business units.
Challenges and Future Directions
Computational Complexity
One of the main challenges of RAG is its computational complexity. Integrating retrieval and generation requires significant computational resources, which can be a barrier for some enterprises. However, ongoing advancements in hardware and optimization techniques are likely to mitigate this issue over time.
Data Quality and Availability
The effectiveness of a RAG system depends heavily on the quality and availability of the underlying data. Ensuring that the retriever has access to high-quality, relevant information is crucial for the success of the system. As more high-quality datasets become available, the performance of RAG systems is expected to improve.
Ethical Considerations
Like all AI technologies, RAG raises important ethical considerations. Ensuring that the generated content is unbiased, fair, and ethical is crucial. Developers must implement robust safeguards to prevent the dissemination of misinformation or harmful content.
Integration with Existing Systems
Integrating RAG with existing web content management systems (CMS) can be challenging. Enterprises need to ensure that their web CMS can seamlessly integrate with the RAG and AI/ML system. For example, an API-first headless CMS is best for easy integration and creating highly productive AI-based content creation and management workflows.
Example Use Cases: RAG in Action
E-Commerce Product Descriptions
An e-commerce company could implement a RAG system to automate the creation of product descriptions. By leveraging a vast database of product information and user reviews, the RAG system would generate detailed and engaging product descriptions that significantly improve user engagement and conversion rates. The system would also enable the company to quickly update product descriptions based on new information, ensuring that the content was always current and relevant.
Financial Services Personalized Content
A financial services firm can utilize RAG to create personalized content for its website and mobile app users. By retrieving relevant information about each user's financial history and preferences, the RAG system could generate customized investment advice and product recommendations. This personalized approach would not only enhance user satisfaction, but also drive higher engagement and conversion rates.
Tech Company Documentation
A technology company can implement RAG to streamline the creation of technical documentation for its products. By retrieving relevant information from internal knowledge bases and previous documentation, the RAG system could generate accurate and detailed user manuals, API documentation, and troubleshooting guides. This would significantly reduce the time and effort required to produce high-quality documentation, allowing the company to focus on innovation and product development.
Conclusion
Retrieval-Augmented Generation (RAG) represents a significant advancement in the field of machine learning, offering a powerful and flexible approach to enterprise content creation. By enhancing the accuracy and relevance of generated content, RAG can revolutionize the way enterprises create and manage their online presence. From personalized user experiences and dynamic FAQ sections to localized content and streamlined documentation, RAG offers a wide range of applications that can drive efficiency, consistency, and engagement. As the technology continues to evolve, we can expect to see even more innovative and impactful uses of RAG, helping enterprises stay ahead in the competitive digital landscape.
To learn more about how CrafterCMS supports AI-based content creation, read our related blog post: Generative AI Use Cases For B2B Content Teams
Related Posts
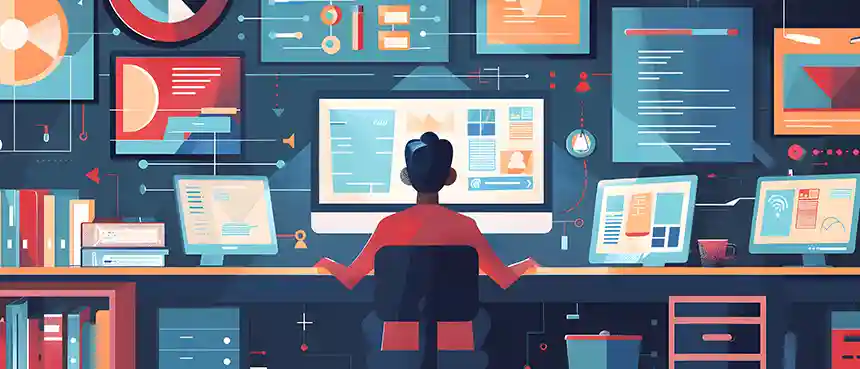
Architected for Scale: Managing 1000s of Sites and Users with CrafterCMS
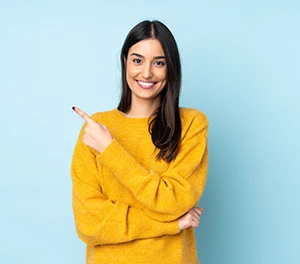
Amanda Jones
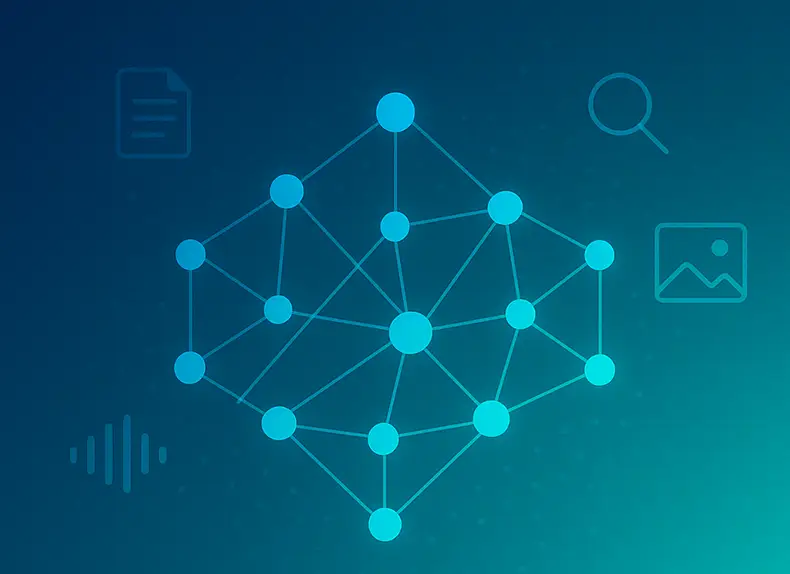
Leveraging OpenSearch for AI-Powered Content Management
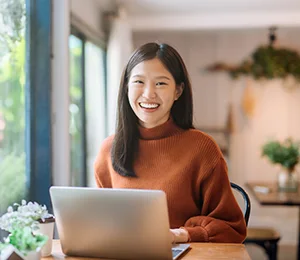
Amanda Lee
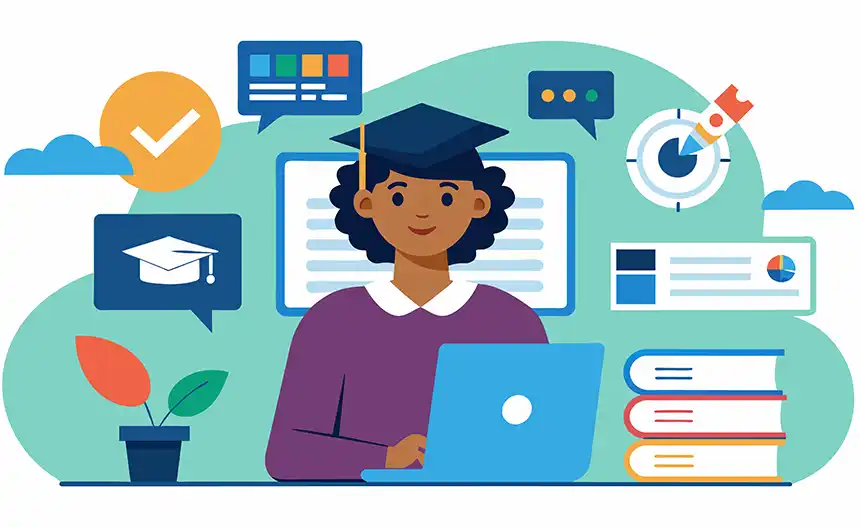
Scaling Digital Experiences in EdTech: How Encoura Standardized Content Management with CrafterCMS
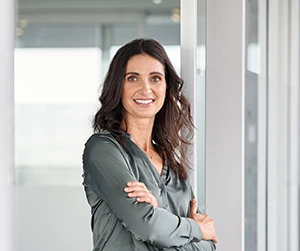
Sara Williams
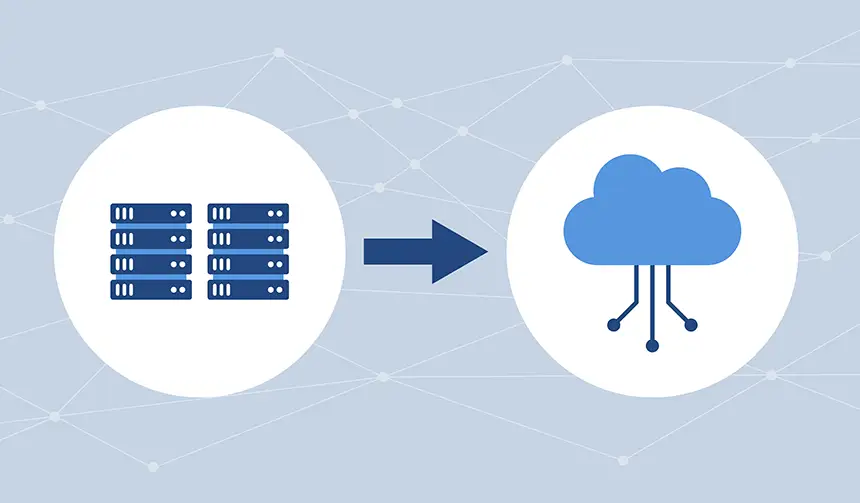
Migrating from OpenText Web CMS (Teamsite): Why Enterprises Are Moving to CrafterCMS
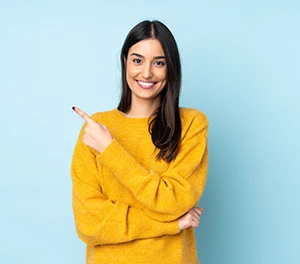
Amanda Jones
Related Resources
-
Personalized Digital Experiences for a Cruise Liner
Webcast
-
How to Migrate from Contentful to CrafterCMS
Tutorial
-
How Content Led e-Commerce Drives Customer Engagement
Webcast
-
Marriott: Streaming Enterprise Video in the Hybrid Cloud
Case Study
-
Dramatically Improving Content Management for Large-Scale Enterprise Websites
Webcast